Data in the Fast Lane
Accelerate Value Creation through Big Data Analytics
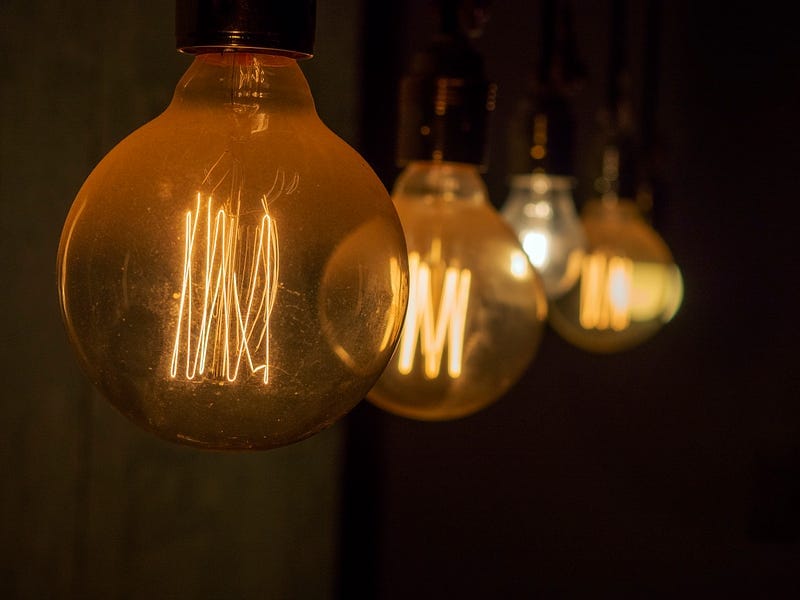
Regardless of where you are in your career journey in Data Science or whichever sector(s) that you are in, the ability to harness the potential of big data analytics and transform it into tangible values has become an indispensable skill, a driving force behind your success and a catalyst for your organization’s growth in today’s data driven landscape.
Companies amass colossal amounts of data daily, but the transformation of this data into tangible value remains a struggle for many. Despite substantial investments in data analytics, the promised insights often remain elusive, trapped within a maze of technology and processes. This age-old challenge persists across countless organizations.
The Dilemma
Over the past two decades, the realm of data science has seen unprecedented growth and innovation, yet surprisingly, the very methods we employ to extract value from data have, for the most part, clung to traditional paradigms that were established years ago.
This lack of evolution becomes even more striking when we consider the rapid pace at which the data landscape has transformed. Our ability to generate, collect, and store data has skyrocketed, thanks to the advent of cloud computing, the Internet of Things (IoT), and the explosion of online interactions. However, the project management methodologies of data analytics projects have lagged behind, leaving us in a paradoxical situation where we possess an abundance of information, yet struggle to fully capitalize on its potential.
This stagnation has far-reaching implications. It has limited our capacity to adapt to new challenges and capitalize on emerging opportunities. It has hindered our ability to make informed decisions swiftly and with confidence. As a result, organizations have found themselves wrestling with data rather than leveraging it as a strategic asset.
The Solution #1: Information Value Chain
The current emphasis in IT development frequently centers on the creation of software applications. However, it’s important to recognize that a truly effective Big Data Analytics (BDA) methodology demands a shift in focus. Centering BDA systems on the information value chain acknowledges that the true power of data lies in its transformation into knowledge and its seamless dissemination to those who can make informed decisions based on it.
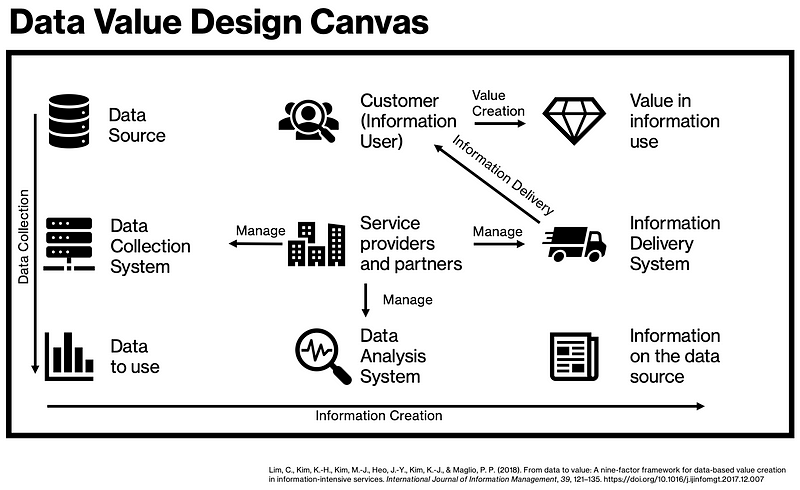
The data value chain or information value chain is a conceptual framework that illustrates the journey of data from its generation and collection to its transformation into valuable outcomes. It emphasizes that the value derived from data is not a one-step process. Instead, it’s a dynamic and interconnected series of stages, where each phase builds upon the previous one.
In the organizational landscape, the data analytics department plays a pivotal role — it operates as a hub of information-intensive service (IIS) providers, catering to the diverse needs of other departments within the organization. Let’s first outline the key building blocks that constitute an information-intensive service:
- Data Source: The quality and relevance of the origin of data (e.g., people, objects) can greatly influence what data can be extracted from it.
- Robust Data Collection System: Data can be collected via physical or social sensing. The infrastructure and methodology in place to gather compile data from various sources must be reliable and efficient to handle the volume, variety and velocity of data being collected.
- Raw Data: Unprocessed and unfiltered data collected from various sources serves as the starting point for any data analysis. It can be structured, semi-structured or unstructured.
- Intricate Process of Data Analyses: Systematic examination of data employs statistical techniques, machine learning algorithms and data visualization tools to identify patterns, trends, correlations and more.
- Insights from Data Analyses: Actionable findings derived from the data analysis process answer the questions posed and provide valuable information that can drive informed decisions. The information can be descriptive, diagnostic, predictive or prescriptive.
- Seamless Information Delivery System: Channels or platforms through which insights are conveyed to end-users can include reports, dashboards, presentations, real-time alerts and more.
- Collaborative Efforts of Service Providers and Partners: It’s important for IIS to work together with data partners and subject matter experts (SMEs) as it fosters diverse perspectives that can lead to more comprehensive insights.
- Information Users: Understanding the needs and preferences of end users (e.g., senior leadership, stakeholders) is crucial to tailor the insights in ways that are easily comprehensible and actionable.
- Inherent Value within the Application of the Information: It’s not just about having the insights but also about leveraging them to either avoid undesirable outcomes or to drive desirable outcomes. Potential positive impact can only be realized when the insights are applied to real-world scenarios.
This comprehensive framework forms a robust foundation that guide us in addressing crucial inquiries in the data value design process:
- What precisely should be delivered?
- Why is this delivery of information significant?
- To whom should this valuable information be directed?
- How should this information be efficiently produced?
- Finally, the logistical considerations of how, when, and where to seamlessly deliver this valuable information to achieve optimal impact.
By thoughtfully examining and addressing these elements, we pave the way for a structured and holistic approach to data value creation, ensuring that each aspect of the information-intensive service aligns harmoniously to drive innovation, informed decision-making, and overall organizational excellence.
Solution #2: Integrate Benefits Realization Management (BRM) with Big Data Analytics (BDA) Systems
When building effective BDA systems, it’s crucial to acknowledge that the true benefits arise from changes within the organization, not merely from the capabilities of the technology itself. This highlights the significance of benefits realization management — an approach that enhances the identification of valuable and attainable benefits, while also ensuring that these benefits are translated into reality through deliberate decisions and proactive actions. In essence, benefits realization management plays a pivotal role in bridging the gap between potential benefits and tangible outcomes.
BRM is usually divided into three phases:
- Identify Benefits: Determine whether projects, programs and portfolios can produce intended business results
- Execute Benefits Management: Minimize risks to future benefits and maximize the opportunity gain additional benefits
- Sustain Benefits: Ensure continued value creation
Unfortunately, this topic is beyond the scope of this article. If you are interested in learning more about how you can seamlessly integrating BDA with BRM and what are the challenges in doing so, please refer to https://aisel.aisnet.org/ecis2019_rp/47/
Solution #3: Tightly Connect Insights with Business Strategy using PADIE Technqiue
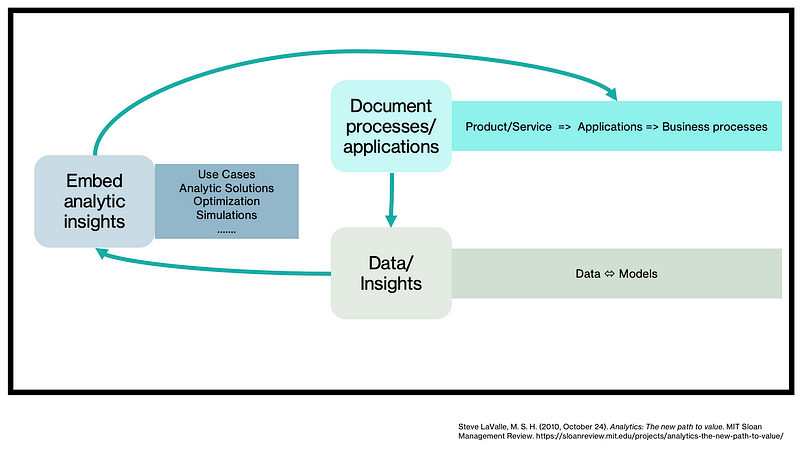
Now, let’s transition our attention to the broader organizational scope.
How can we ignite a ripple of new actions throughout the entire organization by harnessing the potential of insights generated through analytics? To pave the way from insights to tangible value, it’s crucial to tightly connect insights with business strategy. Moreover, we need to ensure that these insights are readily comprehensible to end-users and seamlessly integrated into organizational workflows, empowering the organization to take timely actions that align precisely with its goals.
One technique to operationalize analytics is PADIE (process-application-data-insights-embed). This technique is executed in three steps:
- Document Existing Processes and Applications: Identify the value they deliver to customers, the application they use to drive the business and their core processes, including management systems and metrics, operational and transactional processes and touch points with external parties
- Identify Data and Insights than can Solve Pain Points and Create Value: Identify the questions — who, what, where, when and why and how — that will address pain points and create revenue, cost or margin value (You can refer to the data value design canvas for this)
- Embed the Insights into the Organization’s Operations (e.g., optimization logic added to rules engine, new workflows or simulations to help management understand varying scenarios)
Common Missteps
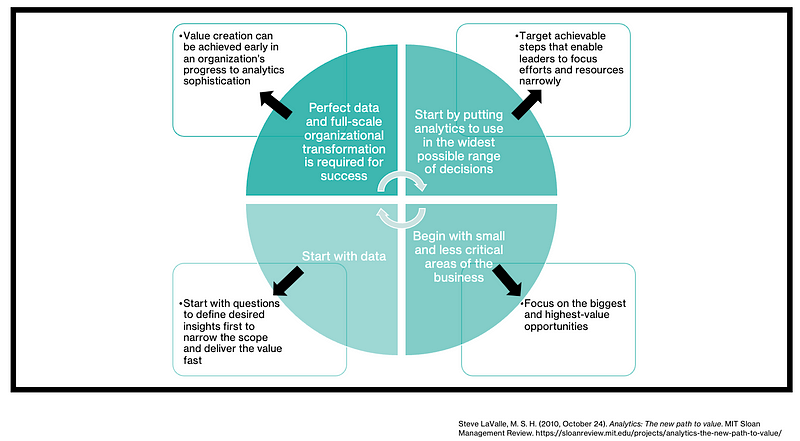
Erroneously, many believe that only perfect data and a full-scale organizational transformation can set us on the path to success. However, the truth is far more encouraging. Organizations can create meaningful value right from the start, even as they are gradually building their analytics capabilities
Another common misconception is that analytics should permeate every corner of our decision-making landscape. Yet, a more effective strategy is to channel our efforts and resources into focused and achievable steps. Organization should concentrate on specific and attainable objectives and a targeted approach ensures analytics efforts yield tangible results.
Some believe that it’s wisest to begin with modest, less critical areas of organizational operations. However, organization should instead focus on the most significant and impactful opportunities. By aligning analytics efforts that require commitments from all sides, we can overcome various challenges, gain executive support, enhance data quality, and foster a culture that embraces data-driven decision-making.
The fourth misconception revolves around beginning our journey with the data itself, which may seem intuitive. Rather than being bogged down by data processes, we should begin with questions that define the desired insights. This narrows the focus and delivers value quickly, without getting stuck in data-related processes. Once insights are delivered, identifying gaps in data, infrastructure, and processes becomes easier.
Conclusion
In closing, by dispelling these common misconceptions and embracing the different approaches, we position ourselves for success in the data-driven era.
Remember, value creation is not bound by perfection or exhaustive organizational transformations; it thrives in the early stages of analytics sophistication. Focused efforts, aligned with strategic objectives, pave the way for impactful results, while prioritizing high-value opportunities propels us towards innovation and growth. By starting with insightful questions rather than drowning in data, we accelerate value delivery and empower timely actions.
Armed with these insights, we can embark on a journey where data becomes a powerful asset, steering our organizations towards informed decisions, optimized processes, and a future illuminated by the endless possibilities of analytics.
References
Saltz, J. S. (2015). “The need for new processes, methodologies and tools to support big data teams and improve big data project effectiveness.” In: Proceedings — 2015 IEEE International Conference on Big Data, IEEE Big Data 2015 (pp. 2066–2071)
Larson, D. and V. Chang. (2016). “A review and future direction of agile, business intelligence, analytics and data science.” International Journal of Information Management, 36(5), 700–710.
Lim, C., Kim, K.-H., Kim, M.-J., Heo, J.-Y., Kim, K.-J., & Maglio, P. P. (2018). From data to value: A nine-factor framework for data-based value creation in information-intensive services. International Journal of Information Management, 39, 121–135. https://doi.org/10.1016/j.ijinfomgt.2017.12.007
Jensen, Maria Hoffmann; Nielsen, Peter Axel; and Persson, John Stouby, (2019). “MANAGING BIG DATA ANALYTICS PROJECTS: THE CHALLENGES OF REALIZING VALUE”. In Proceedings of the 27th European Conference on Information Systems (ECIS), Stockholm & Uppsala, Sweden, June 8–14, 2019. ISBN 978–1–7336325–0–8 Research Papers. https://aisel.aisnet.org/ecis2019_rp/47
Steve LaValle, M. S. H. (2010, October 24). Analytics: The new path to value. MIT Sloan Management Review. https://sloanreview.mit.edu/projects/analytics-the-new-path-to-value/